Integrating AI in Manufacturing: A Guide to Benefits, Challenges, and Practical Solutions
by Nam Le,
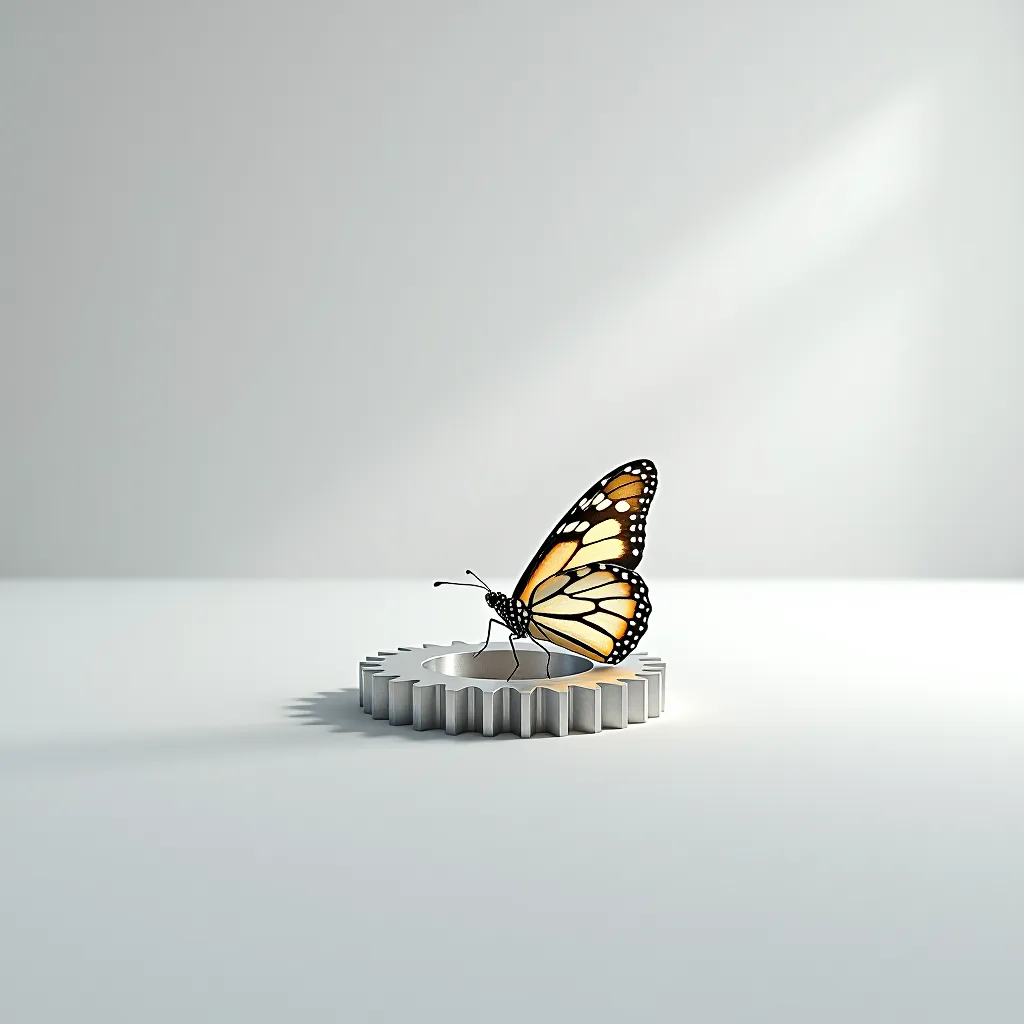
But while countries like China and India, and companies like NVIDIA are leading the charge, the full potential of AI in manufacturing is still largely untapped. The real challenge isn’t in proving AI’s value but in figuring out how to integrate it effectively to harness its true power. Often, within small and large organizations, human adoption presents the biggest hurdles.
The Reality Check on AI Adoption in Manufacturing
It’s clear that AI has the power to redefine manufacturing. Yet, the gap between recognizing its potential and successfully adopting it remains significant. Many manufacturing leaders see the promise of AI but struggle to implement it effectively due to a host of challenges—from integrating with outdated systems to finding the right talent.
This article breaks down emerging AI trends, real-world applications, and practical strategies to help you not only grasp AI’s potential but also deploy it within your operations.
How Can AI Benefit Manufacturing?
AI has the potential to enhance various aspects of manufacturing operations significantly. Here are some key areas where AI can help address challenges and drive improvements:
-
Predictive Maintenance: AI-powered predictive maintenance solutions can analyze sensor data from equipment to predict potential breakdowns and failures before they occur. This allows manufacturers to schedule maintenance and avoid unplanned downtime proactively.
-
Quality Control: Machine vision systems combined with AI can automatically inspect products and detect defects more accurately and consistently than manual inspection. AI can spot deviations from quality standards that may be difficult for humans to identify.
-
Process Optimization: AI algorithms can analyze production data to identify inefficiencies and optimize processes for higher efficiency, productivity, and sustainability. AI can help manufacturers achieve consistent production levels.
-
Supply Chain Optimization: AI can help optimize supply chain operations by forecasting demand, predicting raw material prices, and identifying potential supply chain disruptions. This enables manufacturers to make more informed decisions and improve supply chain resilience.
-
Generative Design: AI-powered generative design tools can rapidly produce hundreds of design options for a product by mimicking the design process used by engineers. This allows manufacturers to explore more innovative designs and optimize for factors like cost, weight, and performance.
-
Workforce Augmentation: AI can assist human workers by automating repetitive tasks, providing real-time insights, and offering guidance and recommendations. This helps workers be more productive and make better decisions.
While these ideas are promising, integrating AI into the manufacturing workflow still seems like a distant goal. This is largely due to the significant challenges that emerging leaders face in realizing AI's potential within their workforce, including:
Challenges with Integrating AI in Industries
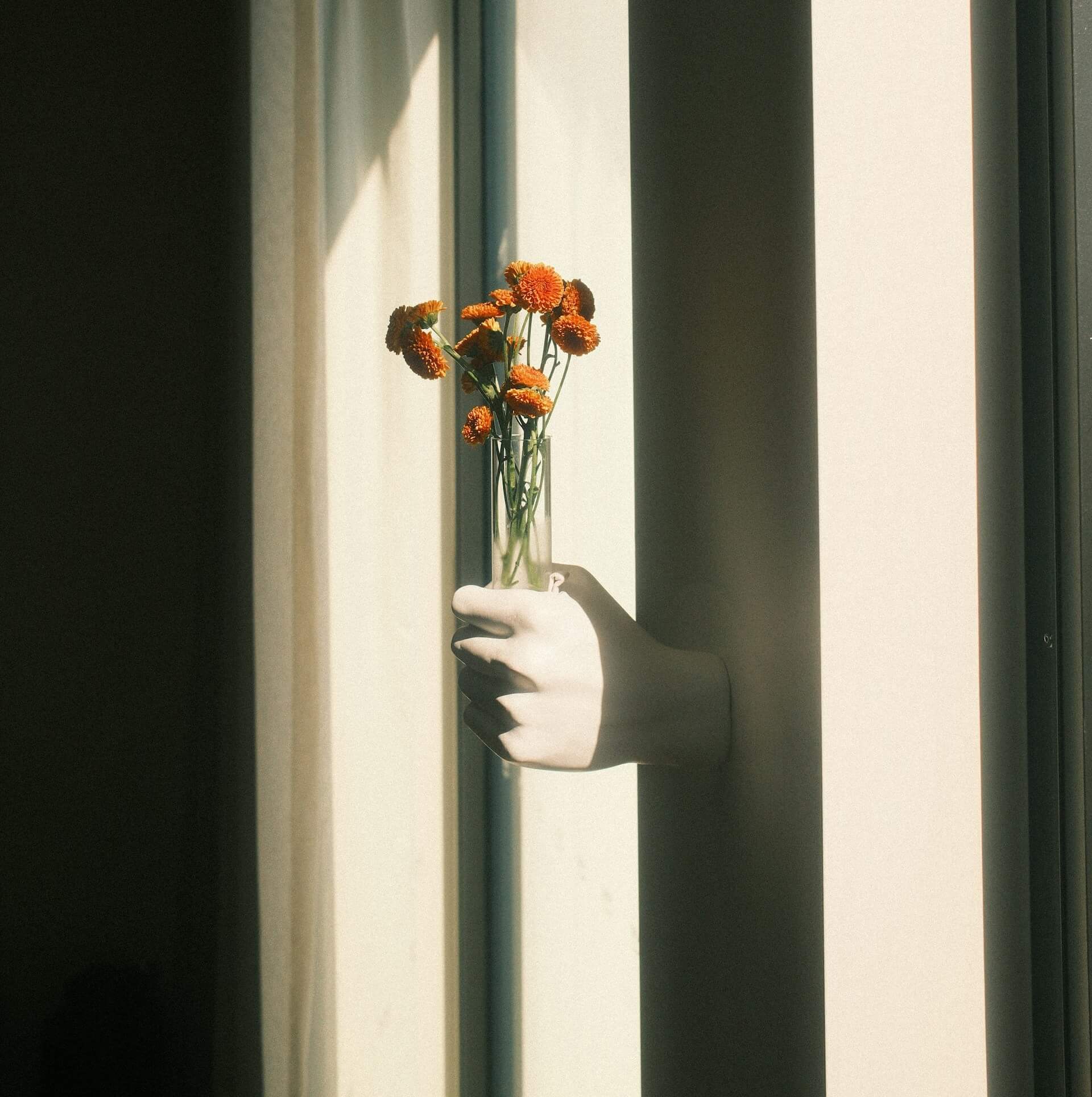
1. Data Collection i.e Quality
AI and machine learning models rely heavily on accessing vast amounts of high-quality data to function effectively. In manufacturing, if the collected data is inconsistent, incomplete, or poorly structured, it can lead to unreliable outputs and hinder decision-making processes.
To address this challenge, it's crucial to precisely define the data requirements for each AI application and ensure that data processing aligns with these needs. Implementing robust data collection and management protocols, including putting a little extra effort toward data collection ways and need so be to make new protocols for it.
2. Scarcity of Skilled Talent
Successfully deploying AI systems in manufacturing requires specialized expertise in areas such as data science, analytics, and automation. The rapid expansion of AI across various industries has led to a competitive landscape for recruiting professionals with these skills, making it challenging for manufacturing companies to build competent AI teams.
To overcome this obstacle, organizations should collaborate with AI integration service providers and industry experts to identify and address specific talent needs. Partnering with capable service providers is the most effective way to avoid challenges related to scarce talent, maintenance, and employee turnover.
3. Integration with Legacy Systems
Many manufacturing firms operate with legacy systems that may not seamlessly integrate with modern AI technologies, posing significant challenges to effective implementation. Incompatibility issues can lead to operational inefficiencies and prevent organizations from fully leveraging AI capabilities.
Addressing integration challenges requires a thorough assessment of existing infrastructure to identify compatibility issues and integration points. Engaging with AI solution providers early in the process can help evaluate the feasibility of integrating new technologies with current systems and determine necessary upgrades or replacements. Adopting a phased implementation approach allows for gradual system enhancements while minimizing disruptions to ongoing operations.
4. Overambitious Implementation Timelines
Leaders eager to rapidly harness the benefits of AI can pursue aggressive implementation schedules. It results in inadequate planning and suboptimal outcomes. Rushed deployments often overlook critical factors, leading to systems that fail to meet organizational expectations.
To ensure successful AI integration, it's important to develop a realistic and structured roadmap that outlines a phased approach to adoption. Starting with pilot projects allows organizations to test, learn, and refine their strategies before scaling up. Focusing on incremental improvements and allocating resources for continuous monitoring and iterative optimization ensures that AI systems evolve effectively over time and deliver sustained value.
Conclusion
AI, as we have seen thus far in this article, has the potential to change manufacturing processes inside out with major advantages and implementation hurdles. Simply adopting the new technology is only one aspect of incorporating AI into your manufacturing workflow; you will need a strategy designed to work with not against it, and which focuses on delivering insights relevant to where you want to go.
When it comes to grasping the nuances and unlocking AI power in full spectrum, seek or hire experts well established in integrated Automated BI with your existing ecosystem. These partners offer the consultancy and strategic knowledge needed to integrate AI perfectly into your functionality, as well as introduce the best means of developing growth and performance in equal capacity. In supercharging AI advantage, working with an experienced partner can extend automotive manufacturers the competitive edge that they need to remain ahead in today's rough-and-tumble manufacturing environment.